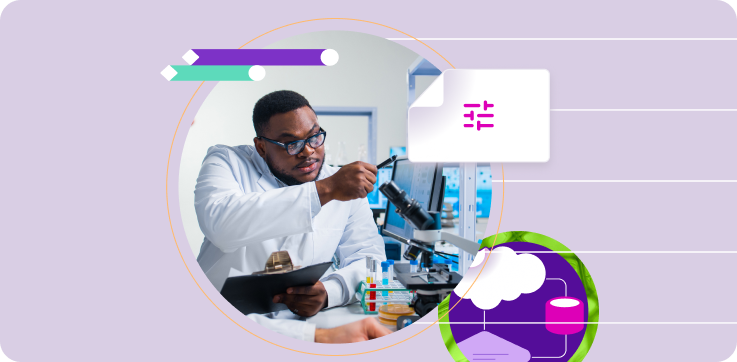
The digital evolution of life sciences has opened the door to the implementation of advanced technologies. They are already revolutionizing how companies develop, manufacture and deliver products into the hands of clinicians and patients.
Today, 45% of life sciences companies’ technology investments are made in three analytics-related technologies – applied AI, industrialized machine learning (ML), and cloud and edge computing.
(McKinsey, Jan 2023) Among MedTech executives surveyed, 91% believe emerging technologies, including cloud and AI, will have a positive impact on their organizations. In a survey of BioPharma leaders, 75% said they plan to invest more in AI in the next five years. (Biopharma digital transformation, Deloitte, 2021)
Predictive AI
With predictive AI, the system uses a manufacturer’s historical data to predict what might happen in the future. For example, algorithms can be trained using historical data to predict when a piece of manufacturing equipment is at risk for failing. In the past, a specific series of events led to the machinery’s failure.
The AI application detects that these events are happening again, makes the correlation, and alerts the system user to this trend.
This individual can intervene to prevent a production disruption or the risk that poor-quality products are produced.
The AI makes the prediction, and the user can act on the information and change reality if they deem the prediction accurate.
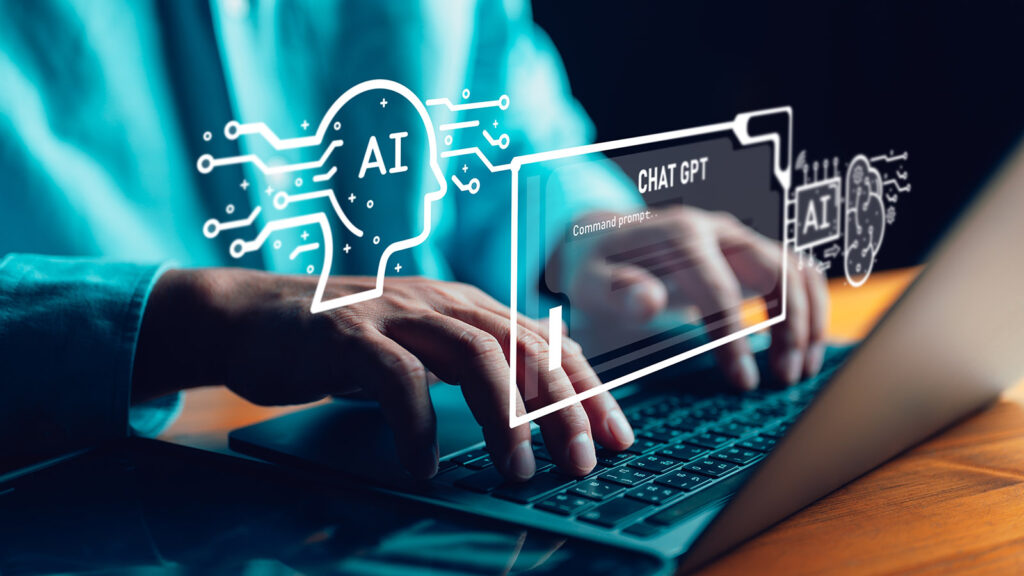
Generative AI
With the introduction of ChatGPT, generative AI (aka ChatBots) has been launched into the spotlight and companies across many industries are working to determine whether they can leverage it to drive value. Falling under the category of machine learning (ML), generative AI is a category of algorithms that generate content (e.g., text, images, audio, video, code) based on existing data sources.
So, unlike predictive AI, which observes and classifies patterns in data, generative AI creates something new from existing data sources.
For example, a user could ask a generative AI model trained on FDA life sciences regulations, “what guidance has the FDA issued with regards to the use of AI in drug development” and the model would return a summary based on the information on which it was trained.
Some life sciences companies have begun experimenting with generative AI to inform research and development (R&D) efforts. For example, Janssen Pharmaceutical, a drug company owned by Johnson & Johnson, is testing Syntegra’s AI generated “synthetic patient data” to “to synthesize a patient journey more adequately” in the absence of real-world data (RWE).
What the near future holds for AI use in quality management systems (QMS)
Generative AI technologies, particularly ChatGBT, are generating tremendous buzz among companies, consumers and the media. While ChatGPT might appear on the surface to be a novelty, use of generative AI holds true potential in life sciences quality management. When leveraged strategically and with focus in an eQMS, it can rapidly deliver into the hands of quality professionals targeted information derived from the analysis of internal and external data.
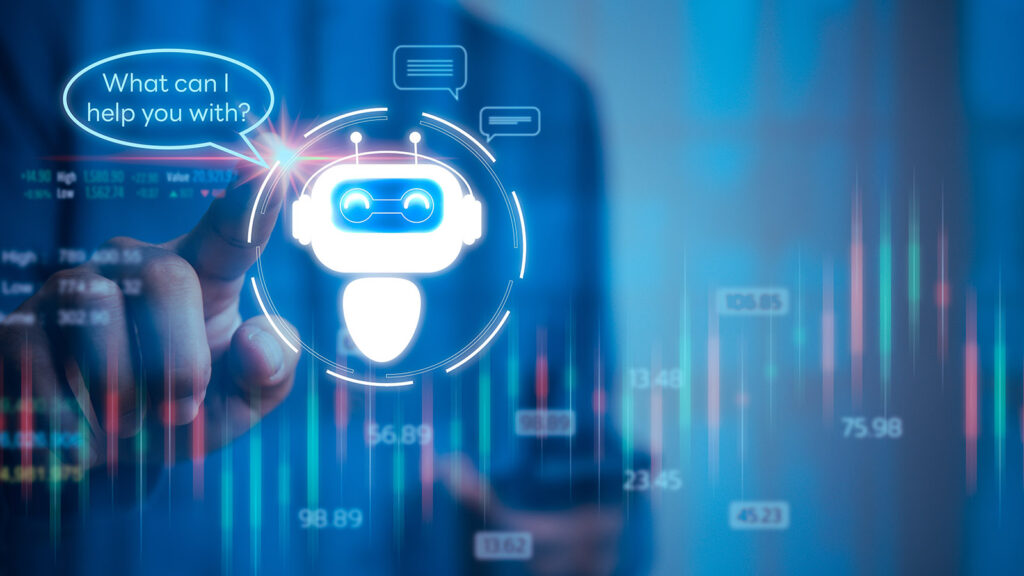
As eQMS solutions have evolved over the years, the data generated and captured has exploded in volume and scope.
A decade ago, a quality management professional with a highly manual and disconnected quality management system might have had access to a handful of data points on their company’s product development or manufacturing processes. Today, a professional with a fully integrated, enterprise-wide cloud based eQMS with digital processes and data likely has hundreds of data points. This volume of data will continue to grow exponentially as technology continues to evolve.
This creates a huge challenge for the life sciences industry. With more data comes more responsibility. A quality manager with one data point they must analyze to assess its impact on product quality and safety to comply with regulations likely has the time and resources to do perform this task. But a manager faced with 100 data points, all requiring investigation, creates a tremendous problem for the company in the modern quality and compliance space.
Generative AI has the potential to provide quality management professionals the power to investigate the impact of data points and trends with the speed and accuracy required in today’s business and regulatory environment. They can sift through the signs to determine where to act based on the nature of the problem/quality issue, and exactly what type of action is required.
Generative AI within an enterprise-wide cloud-based eQMS also enables the quality team to communicate recommended actions to all relevant stakeholders, both internal functions (e.g., R&D, manufacturing, distribution) and outside suppliers. It is the next phase of quality management – real-time information and actionable insights for making strategic decisions with unprecedented speed.